28 Correlation
Correlation is a statistical method used to assess a possible association between two numeric variables, X and Y. There are several statistical coefficients that we can use to quantify correlation depending on the underlying relation of the data. In this chapter, we’ll learn about four correlation coefficients:
- Pearson’s \(r\)
- Spearman’s \(r_{s}\) and and Kendall’s \(\tau\)
- Coefficient \(ξ\)
Pearson’s coefficient measures linear correlation, while the Spearman’s and Kendall’s coefficients compare the ranks of data and measure monotonic associations. The new \(ξ\) correlation coefficient is more appropriate to measure the strength of non-monotonic associations.
When we have finished this Chapter, we should be able to:
28.1 Research question
We consider the data in Birthweight dataset. Let’s say that we want to explore the association between weight (in g) and height (in cm) for a sample of 550 infants of 1 month age.
28.2 Packages we need
We need to load the following packages:
28.3 Preparing the data
We import the data BirthWeight in R:
library(readxl)
BirthWeight <- read_excel(here("data", "BirthWeight.xlsx"))
We inspect the data and the type of variables:
glimpse(BirthWeight)
Rows: 550
Columns: 6
$ weight <dbl> 3950, 4630, 4750, 3920, 4560, 3640, 3550, 4530, 4970, 3740, …
$ height <dbl> 55.5, 57.0, 56.0, 56.0, 55.0, 51.5, 56.0, 57.0, 58.5, 52.0, …
$ headc <dbl> 37.5, 38.5, 38.5, 39.0, 39.5, 34.5, 38.0, 39.7, 39.0, 38.0, …
$ gender <chr> "Female", "Female", "Male", "Male", "Male", "Female", "Femal…
$ education <chr> "tertiary", "tertiary", "year12", "tertiary", "year10", "ter…
$ parity <chr> "2 or more siblings", "Singleton", "2 or more siblings", "On…
The data set BirthWeight has 550 infants of 1 month age (rows) and includes six variables (columns). Both the weight
and height
are numeric (<dbl>
) variables.
28.4 Plot the data
A first step that is usually useful in studying the association between two numeric variables is to prepare a scatter plot of the data. The pattern made by the points plotted on the scatter plot usually suggests the basic nature and strength of the association between two variables.
p <- ggplot(BirthWeight, aes(height, weight)) +
geom_point(color = "blue", size = 2) +
theme_minimal(base_size = 14)
ggMarginal(p, type = "histogram",
xparams = list(fill = 7),
yparams = list(fill = 3))
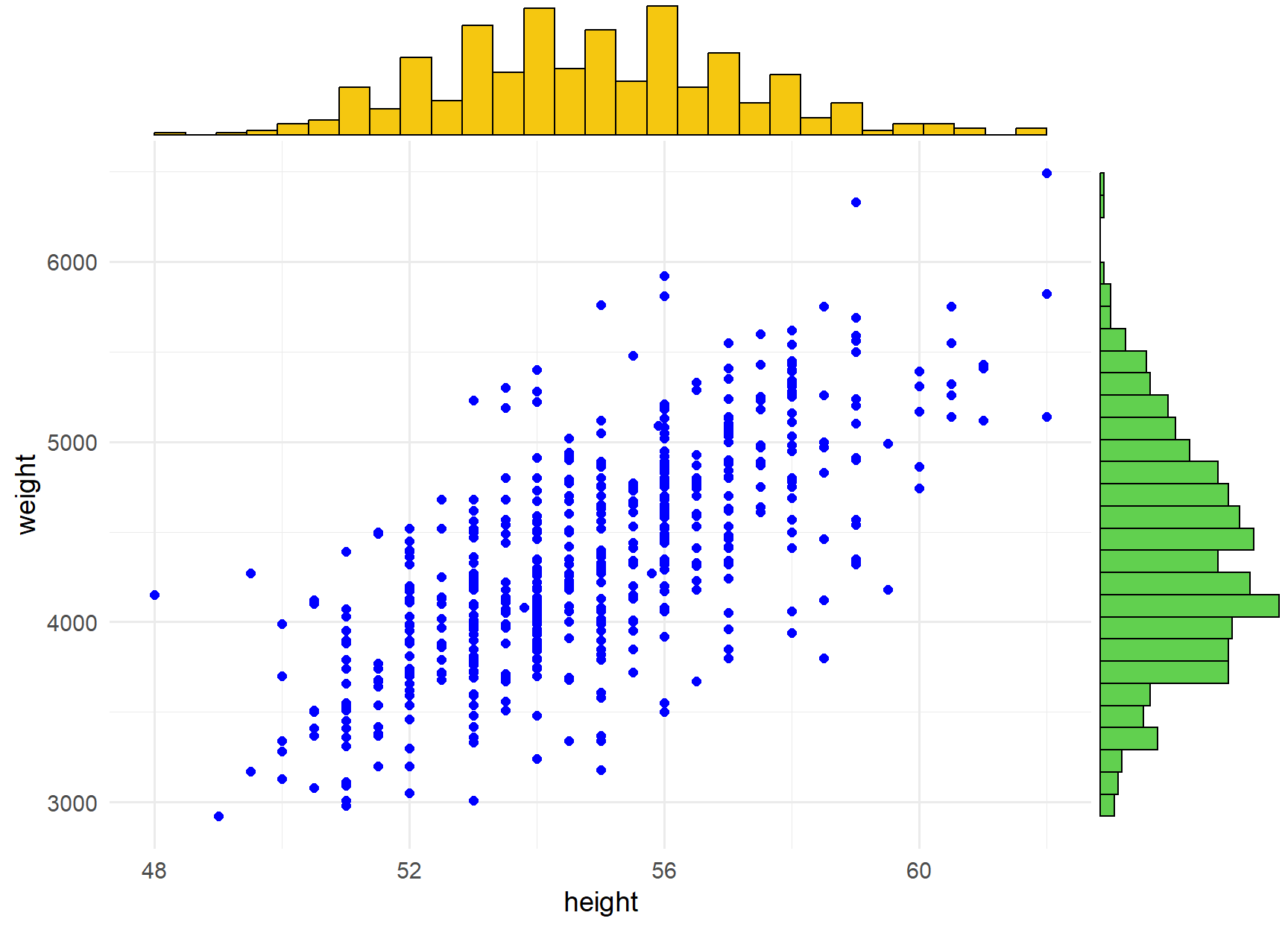
28.5 Linear correlation (Pearson’s coefficient \(r\))
28.5.1 The formula
Given a set of \({n}\) pairs of observations \((x_{1},y_{1}),\ldots ,(x_{n},y_{n})\) with means \(\bar{x}\) and \(\bar{y}\) respectively, \(r\) is defined as:
\[r = \frac{\sum_{i=1}^n (x_i - \bar{x})(y_i - \bar{y})}{\sqrt{\sum_{i=1}^n (x_i - \bar{x})^2 \sum_{i=1}^n(y_i - \bar{y})^2}} \tag{28.1}\]
We observe that the Equation 28.1 is based on calculating the sum of the product \((x_i - \bar{x})(y_i - \bar{y})\). In our example, that is the the sum of the product \((height_{i} - \overline{height}) \cdot (weight_{i} - \overline{weight})\). Our approach begins by examining the signs of these products.
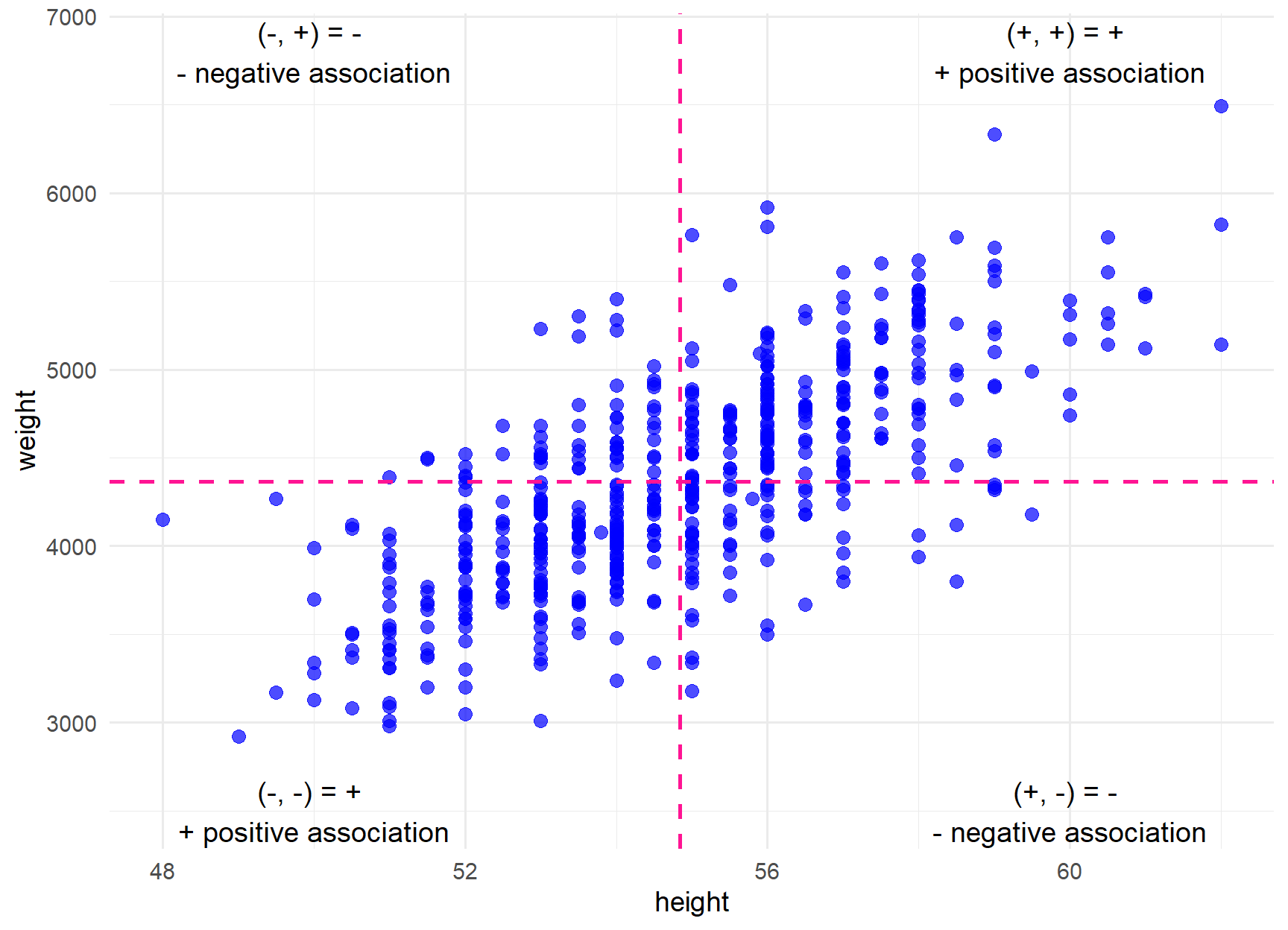
Positive product: In the top-right pane of the Figure 28.3, the deviations from the mean for both variables, height and weight, are positive. Consequently, their products will also be positive. In the bottom-left pane, the deviations from the mean for both variables are negative. Once again, their product will be positive.
Negative product: In the top-left pane of the Figure 28.3, the deviation of height from its mean is negative, while the deviation of weight is positive. Therefore, their product will be negative. Similarly, in the bottom-right pane, the product will be negative.
We observe that most of the products are positive. By applying the Equation 28.1, we can calculate the Pearson’s correlation coefficient, a task that can be easily carried out using R:
cor(BirthWeight$height, BirthWeight$weight)
[1] 0.7131192
The Table 28.1 demonstrates how to interpret the strength of an association according to (Evans 1996).
Value of r | Strength of association |
---|---|
\(|r| \geq{0.8}\) | very strong association |
\(0.6\leq|r| < 0.8\) | strong association |
\(0.4\leq|r| < 0.6\) | moderate association |
\(0.2\leq|r| < 0.4\) | weak association |
\(|r| < 0.2\) | very weak association |
In our example, the coefficient equals r = 0.713, indicating that infants with greater height generally exhibit higher weight. We say that there is a linear positive association between the two variables. However, correlation does not mean causation (Altman and Krzywinski 2015).
Even though summary statistics, such as Pearson r, can provide useful information, they are just simplified representations of the data and may not always capture the full picture. This is typically demonstrated with the Anscombe’s quartet, highlighting the need to explore and understand the underlying patterns and associations within the data through graphical representations (Figure 28.6).
28.5.2 Hypothesis Testing
28.5.3 Assumptions
Before we conduct a statistical test for the Pearson r coefficient, we should make sure that some assumptions are met.
Based on the Figure 28.2 the points seem to be scattered around an invisible line without any important outlier value. Additionally, the marginal histograms show that the data are approximately normally distributed (we have a large sample so the graphs are reliable) for both weight and height. Therefore, we conclude that the assumptions are satisfied.
28.5.4 Run the test
To determine whether to reject the null hypothesis or not, a test is conducted based on the formula:
\[t = \frac{r}{SE_{r}}=\frac{r}{\sqrt{(1-r^2)/(n-2)}} \tag{28.2}\]
where n is the sample size.
For the data in our example, the number of observations are n= 550, r= 0.713 and \(SE_{r}=\sqrt{ \frac{(1-0.713^2)}{(550-2)}}= \sqrt{ \frac{(1-0.5084)}{548}} = \sqrt{\frac{0.4916}{548}}= 0.0299\).
According to Equation 29.17:
\[t = \frac{r}{SE_{r}}= \frac{0.713}{0.0299}= 23.8\]
In this example, the value for the test statistic equals 23.8. Using R, we can find the 95% confidence interval and the corresponding p-value for a two tailed test:
cor.test(BirthWeight$height, BirthWeight$weight) # the default method is "pearson"
Pearson's product-moment correlation
data: BirthWeight$height and BirthWeight$weight
t = 23.813, df = 548, p-value < 2.2e-16
alternative hypothesis: true correlation is not equal to 0
95 percent confidence interval:
0.6694248 0.7518965
sample estimates:
cor
0.7131192
BirthWeight |>
cor_test(height, weight) # the default method is "pearson"
# A tibble: 1 × 8
var1 var2 cor statistic p conf.low conf.high method
<chr> <chr> <dbl> <dbl> <dbl> <dbl> <dbl> <chr>
1 height weight 0.71 23.8 1.40e-86 0.669 0.752 Pearson
The result is significant (p < 0.001) and we reject the null hypothesis.
The significance of correlation is influenced by the size of the sample. With a large sample size, even a weak association may be significant, whereas with a small sample size, even a strong association might or might not be significant.
28.5.5 Present the results
Summary table
BirthWeight |>
cor_test(height, weight) |>
gt() |>
fmt_number(columns = starts_with(c("c", "st", "p")),
decimals = 3)
var1 | var2 | cor | statistic | p | conf.low | conf.high | method |
---|---|---|---|---|---|---|---|
height | weight | 0.710 | 23.813 | 0.000 | 0.669 | 0.752 | Pearson |
Report the results (according to Evans 1996)
Effect sizes were labelled following Evans's (1996) recommendations.
The Pearson's product-moment correlation between BirthWeight$height and
BirthWeight$weight is positive, statistically significant, and strong (r =
0.71, 95% CI [0.67, 0.75], t(548) = 23.81, p < .001)
We can use the above information to write up a final report:
We observed a strong, positive linear association between height and weight of one-month-old infants which is significant (Pearson r = 0.71, 95% CI [0.67, 0.75], n = 550, p < 0.001).
28.6 Rank correlation (Spearman’s \(r_{s}\) and Kendall’s \(\tau\) coefficients)
Spearman’s correlation \(r_{s}\) and Kendall’s coefficient \(\tau\) are both non-parametric correlation coefficients used to measure the strength and direction of association between two variables. Both coefficients are based on the concept of ranking the data but they employ distinct methods in their calculation and have some different characteristics (Puth, Neuhäuser, and Ruxton 2015).
28.6.1 Hypothesis Testing
28.6.2 Assumptions
28.6.3 Run the test
cor.test(BirthWeight$height, BirthWeight$weight, method = "spearman")
Warning in cor.test.default(BirthWeight$height, BirthWeight$weight, method =
"spearman"): Cannot compute exact p-value with ties
Spearman's rank correlation rho
data: BirthWeight$height and BirthWeight$weight
S = 8013119, p-value < 2.2e-16
alternative hypothesis: true rho is not equal to 0
sample estimates:
rho
0.711021
BirthWeight |>
cor_test(height, weight, method = "spearman")
# A tibble: 1 × 6
var1 var2 cor statistic p method
<chr> <chr> <dbl> <dbl> <dbl> <chr>
1 height weight 0.71 8013119. 7.4e-86 Spearman
cor.test(BirthWeight$height, BirthWeight$weight, method = "kendall")
Kendall's rank correlation tau
data: BirthWeight$height and BirthWeight$weight
z = 18.359, p-value < 2.2e-16
alternative hypothesis: true tau is not equal to 0
sample estimates:
tau
0.5408389
BirthWeight |>
cor_test(height, weight, method = "kendall")
# A tibble: 1 × 6
var1 var2 cor statistic p method
<chr> <chr> <dbl> <dbl> <dbl> <chr>
1 height weight 0.54 18.4 2.78e-75 Kendall
We observe that Kendall’s \(\tau\) is smaller than Spearman’s \(r_s\) correlation (0.54 vs 0.71).
28.6.4 Present the results for Spearman’s correlation test
Summary table
BirthWeight |>
cor_test(height, weight, method = "spearman") |>
gt() |>
fmt_number(columns = starts_with(c("c", "p")),
decimals = 3)
var1 | var2 | cor | statistic | p | method |
---|---|---|---|---|---|
height | weight | 0.710 | 8013119 | 0.000 | Spearman |
Report the results (according to Evans 1996)
Warning in cor.test.default(BirthWeight$height, BirthWeight$weight, method =
"spearman"): Cannot compute exact p-value with ties
Effect sizes were labelled following Evans's (1996) recommendations.
The Spearman's rank correlation rho between BirthWeight$height and
BirthWeight$weight is positive, statistically significant, and strong (rho =
0.71, S = 8.01e+06, p < .001)
We can use the above information to write up a final report:
We observed a strong, positive monotonic association between height and weight of one-month-old infants which is significant (Spearman \(r_s\) = 0.71, n = 550, p < 0.001).
28.6.5 Present the results for Kendall’s correlation test
Summary table
BirthWeight |>
cor_test(height, weight, method = "kendall") |>
gt() |>
fmt_number(columns = starts_with(c("c", "st", "p")),
decimals = 3)
var1 | var2 | cor | statistic | p | method |
---|---|---|---|---|---|
height | weight | 0.540 | 18.359 | 0.000 | Kendall |
Report the results (according to Evans 1996)
Effect sizes were labelled following Evans's (1996) recommendations.
The Kendall's rank correlation tau between BirthWeight$height and
BirthWeight$weight is positive, statistically significant, and moderate (tau =
0.54, z = 18.36, p < .001)
We can use the above information to write up a final report:
We observed a moderate, positive monotonic association between height and weight of one-month-old infants which is significant (Kendall \(\tau\) = 0.54, n = 550, p < 0.001).
28.7 Non-monotonic association (coefficient \(ξ\))
The correlation coefficient \(ξ\) ranges from 0 to 1 and is a measure of dependence between X and Y variables (Chatterjee 2021). It equals 1 when the Y is a function of X and it equals 0 when X and Y are independent. Thus, \(ξ\) gives a measure of the strength of the association and it can be used for non-monotonic associations. However, for monotonic associations, it does not indicate the direction of the association.
28.7.1 Hypothesis Testing
28.7.2 Assumptions
28.7.3 Run the test
xicor(BirthWeight$height, BirthWeight$weight, pvalue = TRUE)
$xi
[1] 0.3163988
$sd
[1] 0.02697177
$pval
[1] 0
28.7.4 Present the results
Based on the \(ξ\) correlation coefficient, there is a significant association between height and weight (\(ξ\) = 0.31, sd = 0.027, p < 0.001).